Selection of relevant information to improve Image Classification using Bag of Visual Words
Article Sidebar
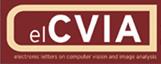
Main Article Content
One of the main challenges in computer vision is image classification. Nowadays the number of images increases exponentially every day; therefore, it is important to classify them in a reliable way.
The conventional image classification pipeline usually consists on extracting local image features, encoding them as a feature vector and classify them using a previously created model. With regards to feature codification, the Bag of Words model and its extensions, such as pyramid matching and weighted schemes, have achieved quite good results and have become the state of the art methods.
The process as mentioned above is not perfect and computers, as well as humans, may make mistakes in any of the steps, causing a performance drop in classification. Some of the primary sources of error on large-scale image classification are the presence of multiple objects in the image, small or very thin objects, incorrect annotations or fine-grained recognition tasks among others.
Based on those problems and the steps of a typical image classification pipeline, the motivation of this PhD thesis was to provide some guidelines to improve the quality of the extracted features to obtain better classification results. The contributions of the PhD thesis demonstrated how a good feature selection can contribute to improving the fine-grained classification, and that there would even be no need to have a big training data set to learn the key features of each class and to predict with good results.
Article Details
Eduardo Fidalgo Fernández, Universidad de León
Dep. of Elect., Systems and Automatic Engineering
Researcher and Coordinator of VARP Group of University of León in INCIBE (Spanish National Institute of Cybersecurity)