Combining Total Variation and Nonlocal Means Regularization for Edge Preserving Image Deconvolution
Article Sidebar
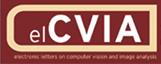
Main Article Content
Binbin Hao
Jianguang Zhu
We propose a new edge preserving image deconvolution model by combining total variation and nonlocal means regularization. Natural images exhibit an high degree of redundancy. Using this redundancy, the nonlocal means regularization strategy is a good technique for detail preserving image restoration. In order to further improve the visual quality of the nonlocal means based algorithm, total variation is introduced to the model to better preserve edges. Then an efficient alternating minimization procedure is used to solve the model. Numerical experiments illustrate the effectiveness of the proposed algorithm.
Article Details
Com citar
Hao, Binbin; and Zhu, Jianguang. “Combining Total Variation and Nonlocal Means Regularization for Edge Preserving Image Deconvolution”. ELCVIA: electronic letters on computer vision and image analysis, vol.VOL 10, no. 1, pp. 42-53, https://raco.cat/index.php/ELCVIA/article/view/250103.