Fully Convolutional Networks for Text Understanding in Scene Images
Article Sidebar
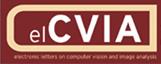
Main Article Content
Dena Bazazian
Text understanding in scene images has gained plenty of attention in the computer vision community and it is an important task in many applications as text carries semantically rich information about scene content and context. For instance, reading text in a scene can be applied to autonomous driving, scene understanding or assisting visually impaired people. The general aim of scene text understanding is to localize and recognize text in scene images. Text regions are first localized in the original image by a trained detector model and afterwards fed into a recognition module. The tasks of localization and recognition are highly correlated since an inaccurate localization can affect the recognition task. The main purpose of this thesis is to devise efficient methods for scene text understanding. We investigate how the latest results on deep learning can advance text understanding pipelines. Recently, Fully Convolutional Networks (FCNs) and derived methods have achieved a significant performance on semantic segmentation and pixel level classification tasks. Therefore, we took benefit of the strengths of FCN approaches in order to detect and recognize text in natural scenes images.
Article Details
Com citar
Bazazian, Dena. “Fully Convolutional Networks for Text Understanding in Scene Images”. ELCVIA: electronic letters on computer vision and image analysis, vol.VOL 18, no. 2, pp. 6-10, https://raco.cat/index.php/ELCVIA/article/view/364072.