Development of transition region based methods for image segmentation
Article Sidebar
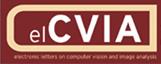
Main Article Content
In this thesis, some transition region based segmentation approaches have developed to perform image segmentation for grayscale and colour images.
In computer vision and image understanding applications, image segmentation is an important pre-processing step. The main goal of the segmentation process is the separation of foreground region from background region. Based on the output of the segmentation result, segmentation can be categorized as global segmentation or local segmentation. The global segmentation aims for complete separation of the object from the background while the local segmentation divides the image into constituent regions. For achieving segmentation, a number of algorithms are developed by various researchers. The segmentation approaches are application specific and do not work well for both grayscale and colour image segmentation. For any image consisting of foreground and background, some transition regions exist between the foreground and background regions. Effective extraction of transition region leads to a better segmentation result. Therefore, the doctoral thesis intends to efficient and effective transition region approaches for image segmentation for both grayscale and colour images.
The performance of the segmentation is qualitatively measured visually by looking at the ground truths as well as the segmentation masks generated from different segmentation approaches or by comparing the original image and the segmented result. The quantitative performance of segmentation results is compared via five performance measures as misclassification error (ME), false positive rate (FPR), false negative rate (FNR), Jaccard index (JI) and segmentation accuracy (SA).
The doctoral research work is focused on the development of transition region approaches both in spatial and wavelet domain for image segmentation. The algorithms developed are categorized as
(A) Grayscale transition region based segmentation approaches
(B) Colour image transition region based segmentation approaches
The details are summarized below.
(A) Grayscale transition region based segmentation approaches: Five transition region based approaches are developed for grayscale image segmentation (i) Proposed method 1, (ii) Proposed method 2, (iii) Proposed method 3, (iv) Proposed method 4 and (iv) Proposed method 5.
(i) Proposed method 1: The Proposed method 1 extracts transition region using local variance and global thresholding considered from the variance features. The method utilizes edge linking and morphological operations for object extraction. The method is intended for segmentation of single and multiple objects from the image. The method does not perform well when the object and background gray level intensities are overlapping in nature.
(ii) Proposed method 2: The Proposed method 2 utilizes 2-dimensional Gabor filter and global thresholding considered from the Gabor features for transition region extraction. Further, it uses edge linking and morphological operations for object extraction. The method is developed for eradicating the drawbacks of Proposed method 1. The performance of this method degrades in the presence of background texture.
(iii) Proposed method 3: The Proposed method 3 is a hybridization of Proposed method 1 and Proposed method 2 in the transform domain. For suppressing the background textures, the wavelet transform is utilized in Proposed method 3. Utilizing the transitional features extracted by applying Proposed method 1 and Proposed method 2, a wavelet feature image is generated. The transition region is extracted by applying Otsu thresholding on these feature image. Further, edge linking and morphological operations are applied on it to extract the objects.
(iv) Proposed method 4: The Proposed method 4 also utilizes the wavelet transform along with uses local standard deviation and Otsu thresholding for transition region extraction. The method suppresses the texture and extracts an effectively continuous transition region achieving better segmentation result. The method does not utilize the edge linking operation as used in Proposed method 1, Proposed method 2 and Proposed method 3. It uses less number of morphological operations as compared to that of Proposed method 1 and Proposed method 2.
(v) Proposed method 5: The Proposed method uses fuzzy c-means clustering of local variance features along with Otsu thresholding for transition region extraction.
(B) Colour image transition region based segmentation approaches: Here three transition region based colour image segmentation which is the extension of gray level transition region approaches are developed. The segmentation methods developed are (i) Proposed method 6, (ii) Proposed method 7 and (iii) Proposed method 8.
(i) Proposed method 6: The Proposed method 6 is an extension of Proposed method 1 developed in RGB colour space.
(ii) Proposed method 7: The Proposed method 7 is an extension of Proposed method 4 processed in CIE-Lab colour space. The method is applied to one agricultural application i.e. worm separation from infected plant leaves.
(iii) Proposed method 8: The Proposed method 8 is a hybrid two-stage segmentation process which uses the algorithm of Proposed method 1 and Proposed method 2. This method processes the colour image in two different colour models (RGB model and CIE-Lab) for object extraction. The Proposed method 8 is applied for underwater fish image segmentation.
The proposed methods are found to be better for segmentation of gray and colour images.Article Details
Articles més llegits del mateix autor/a
- Jyotiprava Dash, Priyadarsan Parida, Nilamani Bhoi, Retinal Blood Vessel Extraction from Fundus Images Using Enhancement Filtering and Clustering , ELCVIA: electronic letters on computer vision and image analysis: Vol. 19 Núm. 1 (2020)
- Priyadarsan Parida, Ranjita Rout, Transition region based approach for skin lesion segmentation , ELCVIA: electronic letters on computer vision and image analysis: Vol. 19 Núm. 1 (2020)